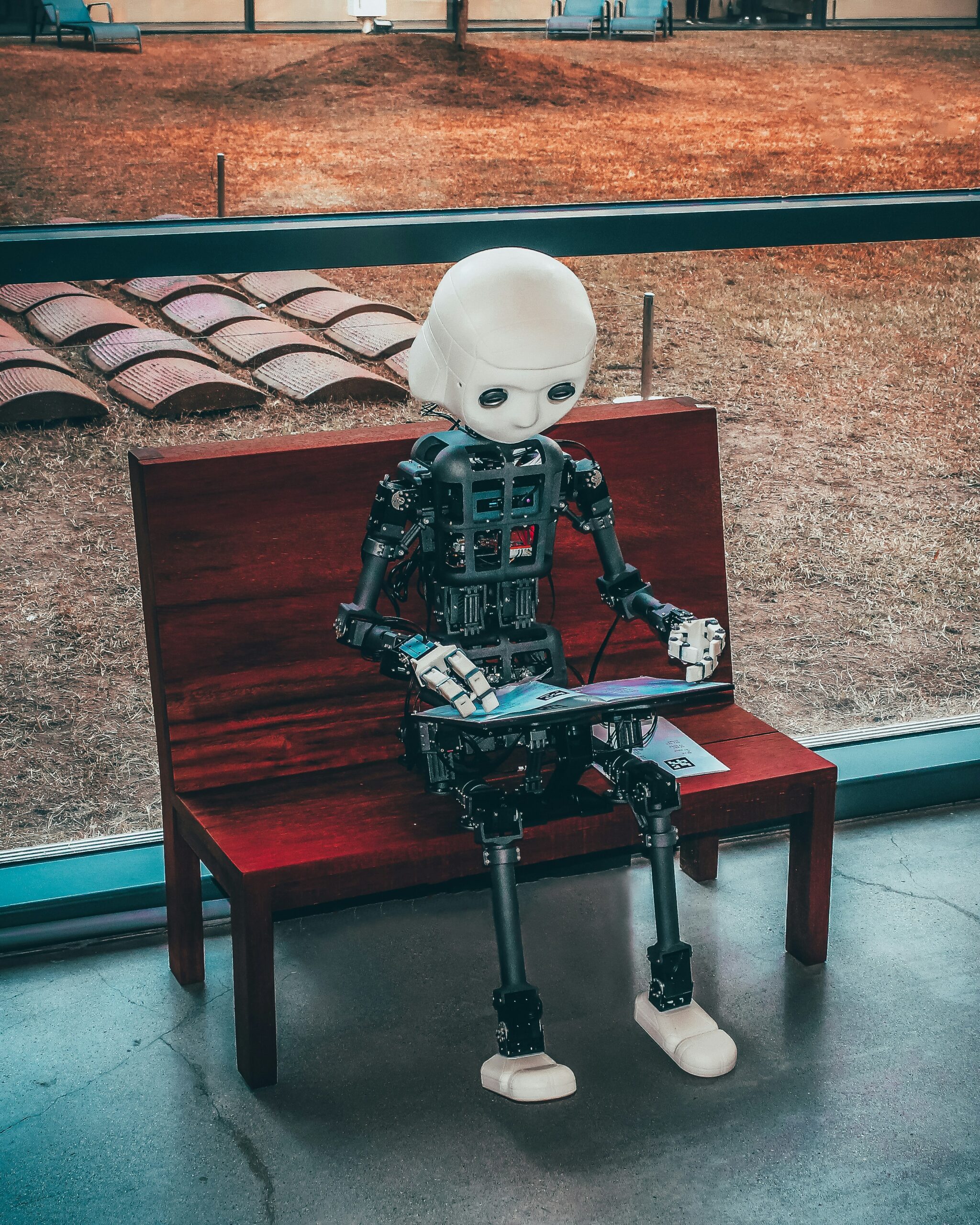
The Evolution of AI Enthusiasm: From Hype to Pragmatism
The advent of large language models (LLMs) and other AI technologies initially sparked immense excitement across the business and technology landscapes. Breakthroughs in natural language processing and machine learning capabilities promised to revolutionize industries, leading to a surge in interest from CIOs and technology leaders. This wave of enthusiasm was fueled by the potential of AI to automate tasks, generate insights from vast amounts of data, and transform customer experiences.
However, as the initial euphoria began to settle, organizations started to grapple with the complexities of implementing AI solutions. It became clear that while AI has transformative potential, it is not a silver bullet. CIOs and decision-makers began to adopt a more pragmatic approach, recognizing that AI must be integrated thoughtfully into existing data engineering and data science frameworks. This transition marked a shift from hype-driven adoption to strategic implementation.
Today, the focus is on leveraging AI as a crucial ingredient that complements and enhances traditional data science efforts. CIOs are prioritizing the alignment of AI initiatives with business goals, ensuring that these technologies deliver measurable value. This includes setting realistic expectations about what AI can achieve and understanding the importance of foundational elements such as robust data infrastructure and governance.
The adoption of AI is now characterized by a more nuanced understanding of its capabilities and limitations. Organizations are investing in upskilling their workforce and developing the necessary infrastructure to support sustainable AI initiatives. This strategic approach is leading to more successful and scalable AI deployments, with CIOs playing a pivotal role in guiding their companies through this evolution.
In summary, the journey from hype to pragmatism in AI adoption underscores the importance of strategic foresight and meticulous planning. By viewing AI as a complementary component rather than a standalone solution, CIOs are better positioned to harness its full potential, driving innovation and delivering long-term value to their organizations.
AI as an Enabler: Enhancing Data Science and Reducing Time to Pilot
In the ever-evolving landscape of data science, Artificial Intelligence (AI) stands out as a transformative force, revolutionizing how organizations approach data-driven initiatives. Crucial to this transformation is AI’s ability to seamlessly integrate into existing data science and data engineering workflows. This integration not only enhances the efficiency of these processes but also significantly reduces the time required to pilot and deploy new projects. By leveraging AI, organizations can experiment and innovate with unprecedented agility.
One of the most compelling advantages of AI is its capacity to automate and streamline complex data science tasks. For instance, AI-powered tools such as automated machine learning (AutoML) platforms enable data scientists to build and deploy models with minimal manual intervention. These platforms expedite the model development lifecycle, from data preprocessing and feature selection to model training and hyperparameter tuning. As a result, organizations can achieve faster time-to-insight, enabling quicker decision-making and more responsive business strategies.
Moreover, AI techniques like natural language processing (NLP) and computer vision are enhancing the accuracy and depth of data analysis. NLP allows for the extraction of valuable insights from unstructured text data, while computer vision automates the interpretation of visual information. These capabilities are particularly beneficial in sectors such as healthcare, finance, and retail, where precise data interpretation is critical.
However, to fully leverage AI’s capabilities, a robust data foundation is essential. Data engineers play a pivotal role in this regard, ensuring that data is clean, structured, and accessible. By implementing best practices in data management and employing advanced tools such as vector databases (vector DB), data engineers create an environment where AI can thrive. Vector DBs, in particular, facilitate the efficient storage and retrieval of high-dimensional data, which is crucial for AI applications.
Successful integration of AI into data strategies requires a strategic approach. CIOs who have excelled in this domain often emphasize the importance of cross-functional collaboration, continuous learning, and iterative development. By fostering a culture of innovation and leveraging AI to its fullest potential, organizations can not only enhance their data science capabilities but also drive sustainable growth and competitive advantage.